Downtime is one of the most costly problems for manufacturers. What if you could reasonably predict when equipment failure will occur based on data collected by IIoT (Industrial Internet of Things) devices?
“Predicting failures via advanced analytics can increase equipment uptime by up to 20%.”
Deloitte
Below, we explain how to implement predictive maintenance analytics from scratch. Maybe you’re vaguely familiar with this term or you may have zero knowledge of the subject. In this post, we show you how predictive maintenance analytics can provide insights that can save you thousands of dollars.
What Is Predictive Maintenance Analytics?
Predictive maintenance analytics helps you predict equipment failure and prevent equipment downtime. It involves comparing real-time monitoring of critical assets and data with historical data to identify potential failure events. This helps prevent breakdowns and minimize maintenance costs.
Predictive maintenance (PdM) is currently the most advanced type of proactive maintenance program. Its predecessors include preventive maintenance and condition-based maintenance (CBM). Out of the two, only CBM uses data and analytics. The technique involves condition monitoring to prevent unplanned downtime.

PdM differs from CBM because PdM uses aggregated sensor data, while CBM relies only on real-time data collected during monitoring.
“Leading executives have used IOT technologies to transform the way their companies service equipment. One of the most popular approaches for doing so in recent years has been the use of predictive-maintenance systems. What could be more attractive than better uptime, greater predictability, and, presumably, lower service costs?”
McKinsey
How Predictive Maintenance Analytics Works
Unlike when scheduling preventive maintenance, you base PdM maintenance schedules on identifying equipment problems that could lead to failure. For this, PdM requires data and an algorithm for data analytics.
Here’s what you need for PdM and how it works:
- Technologies like ML and artificial intelligence (AI): ML and AI algorithms can learn the causes that lead to failure. Predictive maintenance solutions use predictive models powered by these technologies to analyze big data.
- Big data: Historical data helps the machine learning algorithm identify patterns that indicate looming failure. Your IoT sensors will continue to add data to the database. You’ll have to convert this data into structured data. Then, the predictive models can perform data analysis to derive value and context from it.
- Real-time data: Continuously monitoring equipment requires collecting and analyzing real-time sensor data. Predictive models look for patterns and events that can cause failure based on the patterns observed in big data.

Machine Learning for PdM Tools and Analytics
A machine learning model is only as effective as the data you train it with. Therefore, it’s best to work with a data scientist with experience with predictive analytics because you’ll need to cleanse the data you connect before using it.
When collecting training data for your machine learning model, focus on quality.
Data quality is the most critical consideration when collecting data. You’ll need a data scientist to monitor the quality and cleanse the data. Seeking help from a third party for data quality assurance goes a long way.
- The more data you have, the better. When collecting data, you’ll probably wonder when’s a good time to start using the data to train the model. Well, there’s no standard here. But as a general rule, the more data you have, the more accurate predictions your predictive maintenance system will make.
- Select the right data sources. Before collecting data, identify the equipment you want to monitor and the issues you want to avoid. This will help select the correct data sources.
For example, you can use data collected from different components of a welding robot only to predict failures in a welding robot. This is why it’s vital to install sensors on the right equipment.

Data Sources for PdM Analytics
Now that you know what to remember when collecting data, let’s talk about where you can collect data. Below are the types of data sources you can use to collect data. Of course, these aren’t the only data types you can use, but they are the most common ones.
Failure, Repair, and Maintenance Data
Historical data on failure events and maintenance tasks help train the algorithm. Failure events might be rare, but you can use events where you replace spare parts or detect an anomaly as a proxy for failure events.
Maintenance data—including data on repair activities, asset condition before failure, and replaced parts—is critical to train the model. Unfortunately, collecting this data manually is next to impossible. Instead, consider using a computerized maintenance management system (CMMS) for easy data collection. CMMS with robust reporting features will provide you with real-time KPIs and metrics, like MTTB, MTTR, and OEE.

Data from In-Use Equipment
Predictive maintenance assumes that equipment condition degrades as it is in use. Collecting real-time sensor data allows for identifying a specific asset's degradation pattern.
Start by connecting sensors and other monitoring equipment with a CMMS and let the database build over time. Once the algorithm is trained with this data, it can try to look for similar patterns in the data collected in real time.
In addition to identifying anomalies that can lead to failure, algorithms can also use real-time data to predict how long you can use an asset before it requires repairs or you need to replace it.
Metadata
Metadata is a one-and-done data type—you only need to add it to the system once. In addition, metadata includes information like the model, date of manufacture, and technical specifications. These details help the system identify patterns among a narrower category of assets, potentially improving the accuracy of predictions.

Collect Data for PdM Analytics
Data is collected through various types of sensors. The sensors are mounted on the asset strategically to record relevant parameters, such as vibration and temperature. Here are examples of sensors you can use:
- Microelectromechanical sensors (MEMS): MEMS sensors are solid-state accelerometers that collect vibration data. Collecting vibration data can help detect issues related to lubrication, misalignment, etc. Vibration analysis can provide early warnings of machine malfunctioning and prevent downtime.
- Current sensors: Current sensors help prevent equipment damage caused by power spikes. This reduces the cost of repeatedly replacing motors by allowing technicians to troubleshoot the problem.
- Pressure sensors: Pressure sensors help collect data from components used by fluid or gas. The sensors can trigger an alarm when the pressure level breaches a high or low limit.
These are just a few examples of IoT sensors you can install at your plant. These sensors collect data and transmit it to your CMMS software. Maintenance teams can monitor asset performance using this data and, when necessary, address the root cause before the problem leads to failure.
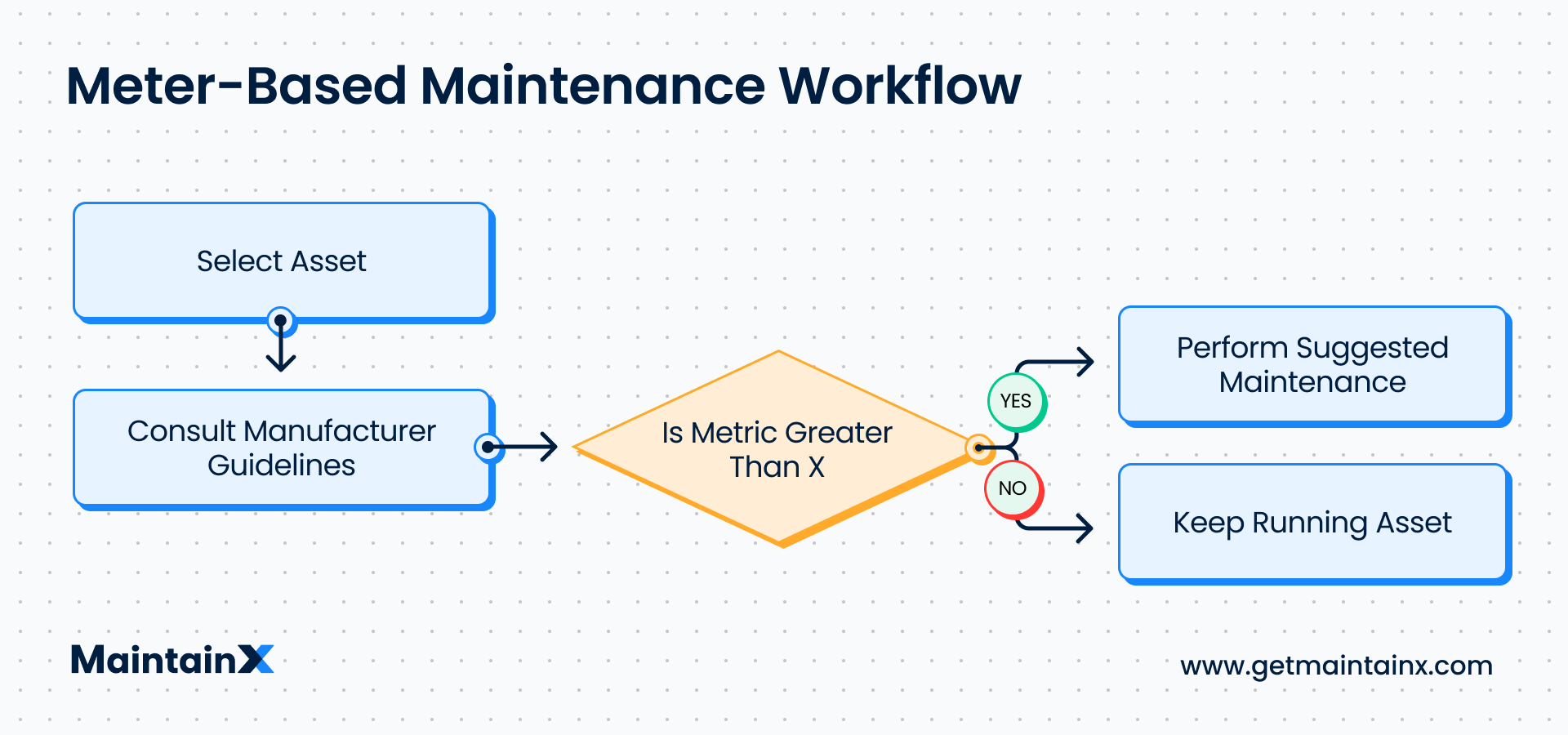
When to Use Predictive Maintenance?
PdM can translate to significant savings when deployed under the right circumstances. However, like all technologies and techniques, predictive maintenance has limitations.
The most noteworthy shortcoming is the possibility of false positives and negatives. These can wipe out the savings PdM helps generate to a great extent.
Deloitte explains that you should reserve predictive maintenance for circumstances with greater risk or lack of predictability.
CBM or advanced troubleshooting (ATS) may yield more cost savings in other situations. Both rely on the same technologies as PdM to extract and analyze data, though they use the data differently than PdM.
So, for most of us, using multiple techniques to reduce downtime makes sense.
Modernize Your Maintenance Strategy with PdM
PdM helps optimize your maintenance activities by facilitating data-driven decisions. It’s important first to assess if investing in a predictive maintenance program will generate an ROI that justifies the investment. Investing in PdM can improve profitability and efficiency if you’re losing a lot of money to asset failure.
Installing sensors is a one-off task, but data collection is ongoing. That’s why having a CMMS to manage data is critical before starting with PdM.
Get MaintainX for Your PDM Program
MaintainX receives data from sensors and automatically adds it to a database. Your PdM model can retrieve data from MaintainX as needed, streamlining the process from data collection to analytics.
MaintainX includes capabilities like scheduled maintenance, work order management, real-time reporting, conditional maintenance scheduling, and parts inventory management. For example, you can create work orders when PdM models suggest an asset requires maintenance using the same data collection app.
Try MaintainX today to see how it can help you streamline maintenance and PdM.
FAQs
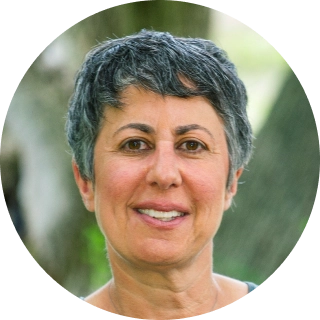
Caroline Eisner
Caroline Eisner is a writer and editor with experience across the profit and nonprofit sectors, government, education, and financial organizations. She has held leadership positions in K16 institutions and has led large-scale digital projects, interactive websites, and a business writing consultancy.